Would You Like To Play a Game (With Chris Williams’ AI Agent)?
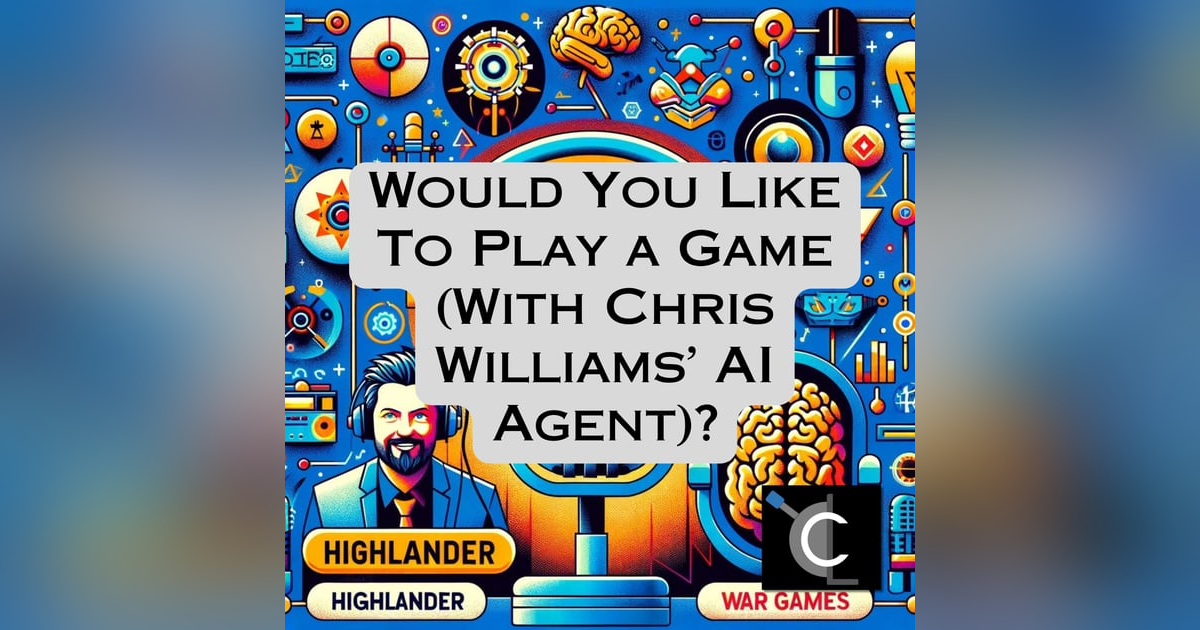
Learn how Chris Williams used ChatGPT to create a custom AI agent that answers questions, completes certain tasks, and Rickrolls its users.
There Can Be Only One (Rickrolling AI Mentor)
Chris Williams joins Ned and Chris this week to discuss the AI agent he recently created using ChatGPT. Ned kicks things off by filling us in on the history of AI from the early 20th century to today. This includes how early AI systems functioned, the role of deep learning models, and the impact of transformers. Chris Williams then explains his use of Retrieval Augmented Generation (RAG) to enhance his AI agent’s answer generation process, the tasks it performs, and its ability to Rickroll users. They also touch on future developments for the AI agent, user feedback, and Chris William’s views on OpenAI’s agent experience.
Links
- Blondie24: Playing at the Edge of AI https://www.goodreads.com/book/show/387996.Blondie24
- Mistwire website: https://mistwire.com/
- DevOps mentor: https://chatgpt.com/g/g-3ZqcfGQQ5-devops-mentor
- LinkedIn: https://www.linkedin.com/in/chrisfwilliams/
00:00:09,080 --> 00:00:11,869
Ned: Hello alleged human, and welcome to the Chaos Lever podcast.
2
00:00:11,900 --> 00:00:14,650
My name is Ned, and I’m definitely not a robot, or a
3
00:00:14,650 --> 00:00:18,639
tuna fish sandwich, or a genetically modified organism.
4
00:00:19,140 --> 00:00:23,220
Because I’m an organism and I have fleshy parts that are true and real.
5
00:00:23,430 --> 00:00:23,980
C Williams: Wink.
6
00:00:24,030 --> 00:00:26,669
Ned: With me as Chris, who’s also here.
7
00:00:26,689 --> 00:00:30,390
And Chris, who’s also—oh, God, I’m in an infinite loop.
8
00:00:30,390 --> 00:00:31,099
D’ah.
9
00:00:31,809 --> 00:00:32,819
Hayner, you go first.
10
00:00:33,309 --> 00:00:33,859
C Hayner: Thank God.
11
00:00:33,860 --> 00:00:34,629
We broke Ned.
12
00:00:34,789 --> 00:00:36,429
Now, we can have an adult conversation.
13
00:00:37,209 --> 00:00:38,220
C Williams: Chris recursion has succeeded.
14
00:00:39,000 --> 00:00:39,030
C Hayner: [laugh]
15
00:00:39,620 --> 00:00:40,819
.
Ned: Just one of the few times.
16
00:00:41,590 --> 00:00:41,640
C Williams: [laugh]
17
00:00:42,920 --> 00:00:43,440
.
Ned: Oh, God.
18
00:00:43,450 --> 00:00:45,580
So, we’re doing something weird here on Chaos Lever.
19
00:00:45,599 --> 00:00:47,380
Well, weirder than usual?
20
00:00:47,790 --> 00:00:48,920
Are you sitting comfortably?
21
00:00:49,210 --> 00:00:50,930
Are your arms and legs inside the car?
22
00:00:50,940 --> 00:00:52,280
Have you gone to the bathroom?
23
00:00:52,959 --> 00:00:53,289
Good.
24
00:00:53,309 --> 00:00:56,519
We have a—wait for it—guest.
25
00:00:57,010 --> 00:00:59,910
For the second time ever, we’ve made the bold decision to bring a guest on
26
00:00:59,960 --> 00:01:04,100
the podcast to talk about something Chris and I are totally clueless about.
27
00:01:04,289 --> 00:01:07,459
And yes, that’s a lot, I acknowledge that, but in this
28
00:01:07,480 --> 00:01:10,039
particular case, we brought on another Chris: Chris Williams.
29
00:01:10,039 --> 00:01:10,620
Hi, Chris.
30
00:01:10,650 --> 00:01:11,619
Welcome to Chao Lever.
31
00:01:11,670 --> 00:01:12,519
C Williams: Holy Spicoli.
32
00:01:12,540 --> 00:01:13,360
Hello, everybody.
33
00:01:13,410 --> 00:01:14,000
How’s it going?
34
00:01:14,370 --> 00:01:16,270
This is Chris Williams, coming to you live.
35
00:01:16,390 --> 00:01:17,070
Allegedly.
36
00:01:17,520 --> 00:01:19,440
Or I could be an AI clone of myself.
37
00:01:19,760 --> 00:01:23,899
Ned: Oh, that is remarkably possible, as we’ll get into shortly.
38
00:01:26,469 --> 00:01:27,070
Chris Hayner.
39
00:01:27,719 --> 00:01:28,009
C Hayner: What?
40
00:01:28,539 --> 00:01:29,229
Ned: How are you?
41
00:01:29,699 --> 00:01:30,530
C Hayner: Why are you asking?
42
00:01:31,000 --> 00:01:31,759
Ned: No reason.
43
00:01:32,380 --> 00:01:34,000
C Hayner: I’m on to you.
44
00:01:34,220 --> 00:01:36,480
C Williams: Such contention in the Chaos Levers?
45
00:01:37,660 --> 00:01:40,159
Ned: [laugh] . Well, it’s because we’re always fighting
46
00:01:40,160 --> 00:01:43,340
over the same thing, which is control of the show [laugh]
47
00:01:43,900 --> 00:01:45,640
.
C Williams: I always want to say Chaos Levers
48
00:01:45,750 --> 00:01:47,929
plural, but it’s Chaos Lever, singular.
49
00:01:48,039 --> 00:01:50,470
Ned: It is singular because there can be only one.
50
00:01:50,930 --> 00:01:52,930
We’re very Highlander in that regard.
51
00:01:53,570 --> 00:01:55,740
C Hayner: In the sense that none of our accents make sense.
52
00:01:56,260 --> 00:01:59,200
Ned: [laugh] . He’s a Spaniard [laugh]
53
00:02:00,190 --> 00:02:01,590
—
C Williams: With a Scottish accent.
54
00:02:02,330 --> 00:02:02,610
Haggis.
55
00:02:03,200 --> 00:02:03,490
What?
56
00:02:04,270 --> 00:02:07,640
Ned: It’s delightful [laugh] . Oh, there’s a segment of
57
00:02:07,640 --> 00:02:10,850
our listeners that really enjoy that exchange, and everyone
58
00:02:10,850 --> 00:02:15,150
else—which I’m figuring is like 98 percent—just nothing.
59
00:02:15,309 --> 00:02:15,769
C Williams: Hi, mom.
60
00:02:16,679 --> 00:02:19,510
Ned: [laugh] . Which means you now have an assignment, everyone who’s listening.
61
00:02:19,510 --> 00:02:22,489
You need to go watch the Highlander movie, maybe
62
00:02:22,490 --> 00:02:26,090
twice to really get it to sink in, and then come back.
63
00:02:26,400 --> 00:02:26,690
And—
64
00:02:26,700 --> 00:02:29,810
C Williams: Do you really have audience members that haven’t seen Highlander?
65
00:02:29,880 --> 00:02:33,979
I mean, in that Venn diagram, it’s pretty much a pure circle, I’m going to say.
66
00:02:34,170 --> 00:02:35,564
Ned: It might be [laugh]
67
00:02:36,229 --> 00:02:36,259
.
C Williams: [laugh]
68
00:02:37,049 --> 00:02:38,440
.
C Hayner: They might have seen them all.
69
00:02:39,330 --> 00:02:42,560
Ned: [laugh] . Oh, so now that we’ve entered hour one of
70
00:02:42,560 --> 00:02:46,250
our six-hour podcast all about Highlander, we will get
71
00:02:46,250 --> 00:02:49,570
into the series, oh yes we will [laugh] . Thank God, no.
72
00:02:50,620 --> 00:02:55,700
Chris Williams, I think you would arise victorious, if only because you have
73
00:02:56,080 --> 00:03:00,570
probably a swo—I’m guessing you have a full sword somewhere in your house.
74
00:03:01,270 --> 00:03:05,040
C Williams: Okay, so because I did a lot of martial arts
75
00:03:05,040 --> 00:03:11,030
growing up, I might have more than one sword in my house.
76
00:03:11,160 --> 00:03:12,189
Ned: And there it is.
77
00:03:12,189 --> 00:03:17,420
Chris only has a swordfish [laugh] and I have a plastic swordfish.
78
00:03:17,430 --> 00:03:19,560
So yeah, we’re going to lose that battle.
79
00:03:20,060 --> 00:03:20,900
C Hayner: It’s not going to go well.
80
00:03:21,150 --> 00:03:23,850
C Williams: To be fair, they are not sharp, and they’re just training swords.
81
00:03:23,950 --> 00:03:28,939
One training Chinese broadsword, one Tai Chi straight sword, and—never mind.
82
00:03:29,020 --> 00:03:29,610
It doesn’t matter.
83
00:03:30,050 --> 00:03:31,356
Ned: So, what you’re saying is you’re going to have to
84
00:03:31,450 --> 00:03:34,310
bludgeon us, as opposed to something quick and painless.
85
00:03:35,410 --> 00:03:36,740
C Williams: [laugh] . It’ll take a while, but I can do it.
86
00:03:38,150 --> 00:03:39,720
Ned: I’m so glad this is over Zoom.
87
00:03:39,950 --> 00:03:43,590
Anyway, we’ve invited you on Chaos Lever to talk
88
00:03:43,600 --> 00:03:46,570
about a thing you built with Python and OpenAI.
89
00:03:46,800 --> 00:03:50,299
But first, we’re going to do some background because that’s what we do.
90
00:03:50,660 --> 00:03:52,889
So, Chris, are you a developer by trade?
91
00:03:52,969 --> 00:03:53,929
Did you use Co—
92
00:03:53,930 --> 00:03:54,540
C Williams: Absolutely not.
93
00:03:54,630 --> 00:03:55,180
Ned: Okay, good.
94
00:03:55,420 --> 00:03:57,680
Did you use Copilot to program the whole damn thing?
95
00:03:57,960 --> 00:03:58,620
C Williams: Actually, no.
96
00:03:59,050 --> 00:03:59,200
Ned: Oh.
97
00:03:59,880 --> 00:04:02,390
How does one grow a beard so luxurious?
98
00:04:02,780 --> 00:04:06,359
C Williams: You get born into the right family, and you just happen
99
00:04:06,370 --> 00:04:09,669
to have the correct genes to make something like this happen.
100
00:04:10,490 --> 00:04:12,810
Ned: Do you moisturize or wax?
101
00:04:12,880 --> 00:04:13,190
No?
102
00:04:14,240 --> 00:04:17,570
C Williams: So many, many years ago, when my wife—my then
103
00:04:17,570 --> 00:04:21,130
girlfriend, now wife—said, “You need to grow a beard.” And she
104
00:04:21,130 --> 00:04:24,489
used to be a professional hairstylist, so I said, “Okay, great.
105
00:04:24,750 --> 00:04:28,089
Does that mean I get stuff like beard oils, and”—because
106
00:04:28,090 --> 00:04:30,230
I’m a gearhead, so I immediately assumed that I was
107
00:04:30,230 --> 00:04:34,299
going to get gearhead stuff aimed at beard owners.
108
00:04:34,690 --> 00:04:35,890
And she was like, “Absolutely not.
109
00:04:36,530 --> 00:04:39,810
Here is the conditioner that you’re going to put in your beard.
110
00:04:39,870 --> 00:04:42,399
Just like this conditioner for your hair that you’d no longer have,
111
00:04:42,420 --> 00:04:45,080
you’re going to now put conditioner in your beard, and that’s it.”
112
00:04:45,089 --> 00:04:50,549
It’s called Verb, and it’s a conditioner, and it’s magically amazing.
113
00:04:50,590 --> 00:04:52,040
She was a hairstylist for 30 years.
114
00:04:52,650 --> 00:04:55,930
She has the inside scoop on all the things, and she said, “Put this in your
115
00:04:55,930 --> 00:04:59,750
beard as you’re washing it, just like you would wash your hair and boom.”
116
00:05:00,500 --> 00:05:04,319
Ned: Wow, I did not expect to get such a thorough answer to my question.
117
00:05:04,750 --> 00:05:06,640
So, I’m going to ask you a stupid question.
118
00:05:06,810 --> 00:05:08,840
Who put the Ram and the Rama Lama Ding Dong?
119
00:05:09,550 --> 00:05:11,390
C Williams: Look, I am here for all the answers.
120
00:05:11,740 --> 00:05:16,049
If you ask me a question I will g—much like my AI clone,
121
00:05:16,300 --> 00:05:19,729
I will give you a nauseatingly verbose answer, regardless.
122
00:05:19,990 --> 00:05:21,370
Ned: [laugh] . I knew it.
123
00:05:23,209 --> 00:05:26,220
Well, I guess before we get into the thing that you built,
124
00:05:26,350 --> 00:05:29,010
maybe we can start with a little historical context for
125
00:05:29,190 --> 00:05:33,280
AI because that’s kind of our thing on the Lever of Chaos.
126
00:05:33,400 --> 00:05:36,450
The current crop of AI tech is using
127
00:05:36,929 --> 00:05:39,760
Generative Pre-trained Transformers, or GPTs.
128
00:05:40,109 --> 00:05:44,380
And I got to be honest, I am as excited as anyone about playing
129
00:05:44,380 --> 00:05:47,780
with transformers, but it’s not that kind of transformer.
130
00:05:48,100 --> 00:05:53,039
Oh, and also, GPT is not even close to the beginning of AI.
131
00:05:53,900 --> 00:05:56,310
AI is not really a new thing.
132
00:05:56,880 --> 00:06:00,760
The earliest ideas of AI were in the late-1950s.
133
00:06:00,760 --> 00:06:04,500
They actually go even further back, but that was around the invention of
134
00:06:04,510 --> 00:06:10,029
the Turing test and the creation of Eliza, the therapist-bot, in the 1960s.
135
00:06:10,340 --> 00:06:11,400
Have you ever seen Eliza?
136
00:06:12,099 --> 00:06:15,680
C Williams: I saw some of the outputs from Eliza, but I never
137
00:06:15,680 --> 00:06:18,129
actually—like, there’s not an emulator for Eliza out there, as
138
00:06:18,129 --> 00:06:20,370
far as I know, so I haven’t—I never actually played with her.
139
00:06:20,760 --> 00:06:21,230
Ned: There is.
140
00:06:21,230 --> 00:06:22,570
If you do a search, you can—
141
00:06:22,570 --> 00:06:23,020
C Williams: Is there really?
142
00:06:23,020 --> 00:06:23,670
Ned: —access one today.
143
00:06:23,680 --> 00:06:23,700
C Williams: Oh, nice.
144
00:06:23,710 --> 00:06:26,780
Ned: And it just uses reflective responses.
145
00:06:27,309 --> 00:06:30,599
So, it just rephrases anything you say in the form of a question.
146
00:06:30,670 --> 00:06:31,100
C Williams: Right, right.
147
00:06:31,270 --> 00:06:34,270
“I’m sad.” “I see that you’re sad, could you tell me more about that?”
148
00:06:34,910 --> 00:06:35,289
C Hayner: My God, he is an
149
00:06:37,800 --> 00:06:37,810
AI.
150
00:06:37,840 --> 00:06:42,750
Ned: But at a certain point, AI kind of hit a wall in the 1970s, and that
151
00:06:42,750 --> 00:06:46,520
wall had a lot more to do with technology than it did with the theory.
152
00:06:47,030 --> 00:06:51,360
Early AI relied on complex rule sets that governed behavior.
153
00:06:51,530 --> 00:06:55,919
If you think of it sort of as a massive decision tree with if-then-else type
154
00:06:55,920 --> 00:07:00,900
logic, that’s extremely deterministic, but it’s not particularly scalable.
155
00:07:01,100 --> 00:07:05,099
So, the concept of Neural Networks, loosely based
156
00:07:05,099 --> 00:07:08,250
on how we think the brain might work, came up.
157
00:07:08,500 --> 00:07:13,510
And we also had model training, and that requires massive parallel computing
158
00:07:13,510 --> 00:07:17,640
to accomplish, but the problem was that computers in the late-1970s
159
00:07:18,090 --> 00:07:21,400
didn’t really have the horsepower required to run the models at any kind
160
00:07:21,400 --> 00:07:27,000
of scale, unless you own the supercomputer, which most of us didn’t.
161
00:07:28,130 --> 00:07:29,689
Though I really wanted the WOPR.
162
00:07:29,799 --> 00:07:33,200
I wanted the WOPR so badly in 1986.
163
00:07:33,410 --> 00:07:34,390
C Williams: “Would you like to play a game?”
164
00:07:34,780 --> 00:07:35,800
Ned: I would love to play a game, Chris.
165
00:07:35,830 --> 00:07:39,060
C Williams: Man, two—two—references in one show?
166
00:07:39,070 --> 00:07:40,189
Hold on to your hats, folks.
167
00:07:40,190 --> 00:07:41,100
This is going to be amazing.
168
00:07:41,360 --> 00:07:44,809
Ned: Oh God, this is just the beginning [laugh] . If
169
00:07:44,809 --> 00:07:47,170
anybody hasn’t seen War Games, they probably should.
170
00:07:47,330 --> 00:07:48,120
C Williams: Start the list.
171
00:07:48,330 --> 00:07:49,409
Highlander, War Games.
172
00:07:49,640 --> 00:07:51,160
Ned: If for no other reason just to see
173
00:07:51,160 --> 00:07:53,090
how young Matthew Broderick looks [laugh]
174
00:07:53,560 --> 00:07:55,310
.
C Williams: I loved the cradle modem, where
175
00:07:55,310 --> 00:07:57,300
you put the phone into the modem interface.
176
00:07:58,620 --> 00:08:00,540
That was my first modem that I had when I was a kid.
177
00:08:00,960 --> 00:08:01,180
C Hayner: Yeah.
178
00:08:01,180 --> 00:08:03,650
The young people probably think that was just a prop.
179
00:08:04,369 --> 00:08:04,589
No.
180
00:08:05,289 --> 00:08:06,860
No, that was actually a thing.
181
00:08:07,210 --> 00:08:08,020
Ned: Sure was.
182
00:08:08,170 --> 00:08:09,410
C Williams: Of course, I can get to the internet.
183
00:08:09,410 --> 00:08:10,190
I do it this way.
184
00:08:10,230 --> 00:08:10,880
Beep boop beep.
185
00:08:11,000 --> 00:08:11,240
Done.
186
00:08:12,190 --> 00:08:15,010
Ned: Most people, yeah, they don’t remember that, but we do.
187
00:08:15,349 --> 00:08:15,909
Yay.
188
00:08:16,170 --> 00:08:17,830
Isn’t getting old awesome?
189
00:08:18,460 --> 00:08:19,190
Fortunately—
190
00:08:21,130 --> 00:08:21,159
C Williams: [laugh]
191
00:08:21,170 --> 00:08:23,020
.
Ned: No, I’m going to—I’m just going to steamroll you right
192
00:08:25,729 --> 00:08:25,847
past there [laugh]
193
00:08:25,847 --> 00:08:25,895
.
C Williams: [laugh]
194
00:08:25,895 --> 00:08:25,943
.
Ned: Fortunately—
195
00:08:25,943 --> 00:08:26,790
C Williams: What kind of show is this?
196
00:08:27,350 --> 00:08:29,310
Ned: Moore’s law is a thing—so it’s a show
197
00:08:29,310 --> 00:08:30,789
where we don’t respect our guests [laugh]
198
00:08:31,010 --> 00:08:31,740
.
C Williams: Absolutely not.
199
00:08:31,740 --> 00:08:32,120
Good.
200
00:08:32,200 --> 00:08:32,450
Good.
201
00:08:32,700 --> 00:08:33,519
I feel right at home now.
202
00:08:33,799 --> 00:08:34,110
Ned: All right.
203
00:08:34,120 --> 00:08:36,020
So, Moore’s law, it’s kind of a thing.
204
00:08:36,049 --> 00:08:40,839
Our processors kept getting denser and faster, and by the late-1990s, we
205
00:08:40,840 --> 00:08:44,150
had the raw horsepower to start doing really interesting things with AI.
206
00:08:44,920 --> 00:08:48,220
And you had stuff like Deep Blue beating Garry Kasparov.
207
00:08:48,570 --> 00:08:50,100
I can see Chris already—
208
00:08:50,280 --> 00:08:51,090
C Hayner: Deep Blue cheated.
209
00:08:51,210 --> 00:08:52,089
Everybody knows it.
210
00:08:52,190 --> 00:08:53,650
Ned: It cheated the first time.
211
00:08:53,910 --> 00:08:54,610
No doubt.
212
00:08:55,059 --> 00:08:58,569
Eventually, it was able to beat Garry Kasparov on its own.
213
00:08:59,100 --> 00:09:00,439
C Hayner: And everyone else.
214
00:09:00,750 --> 00:09:02,800
Well, I mean, that got more complicated, but—
215
00:09:03,250 --> 00:09:03,810
Ned: Yes.
216
00:09:04,260 --> 00:09:05,969
Did you know checkers is a solved game?
217
00:09:06,280 --> 00:09:06,670
C Hayner: Yes.
218
00:09:06,700 --> 00:09:07,560
Everyone knows that.
219
00:09:07,860 --> 00:09:08,973
Ned: No, not everyone knows that.
220
00:09:09,020 --> 00:09:10,980
C Hayner: So, if people are actually interested in hearing about
221
00:09:10,980 --> 00:09:14,759
the history of some of this stuff, there is a book written about
222
00:09:14,759 --> 00:09:18,170
neural networks that were used to create the first AI bot, for
223
00:09:18,170 --> 00:09:21,240
lack of a better term, that could beat a human being at checkers.
224
00:09:21,930 --> 00:09:24,970
It’s at a much lower level of complexity than the ones that, like
225
00:09:24,970 --> 00:09:28,929
Deep Blue play games like chess, which are orders of magnitude more
226
00:09:28,929 --> 00:09:32,850
difficult, but in terms of getting into the space, it’s an awesome book.
227
00:09:32,900 --> 00:09:34,529
I’ve recommended it before on the show.
228
00:09:34,590 --> 00:09:35,650
I’ll recommend it again.
229
00:09:35,930 --> 00:09:38,480
And I can’t say it right now because I’ve also forgotten its name.
230
00:09:38,910 --> 00:09:39,110
Ned: Yep.
231
00:09:39,280 --> 00:09:41,660
[laugh] . Some of the best recommendations, really.
232
00:09:41,660 --> 00:09:42,740
C Williams: That book sounds fantastic.
233
00:09:42,740 --> 00:09:43,890
I can’t wait to purchase it.
234
00:09:44,030 --> 00:09:45,400
C Hayner: Link in the [show notes] , eventually.
235
00:09:45,520 --> 00:09:46,049
Ned: Of course.
236
00:09:46,320 --> 00:09:47,070
If we remember.
237
00:09:47,599 --> 00:09:48,160
C Hayner: Remember what?
238
00:09:48,700 --> 00:09:49,330
Ned: Moving on.
239
00:09:50,790 --> 00:09:53,810
We eventually got into the current millennium, and someone realized that
240
00:09:53,810 --> 00:09:58,430
we could use graphics processing units in high-end graphics cards to do the
241
00:09:58,430 --> 00:10:03,769
parallel processing because AI is really just number crunching done at scale.
242
00:10:04,010 --> 00:10:05,249
It’s a lot of vector math.
243
00:10:05,730 --> 00:10:11,459
And GPUs, they’re really good at doing a specific type of math to render pixels.
244
00:10:11,969 --> 00:10:13,390
That is also vector math.
245
00:10:13,740 --> 00:10:14,320
Yay.
246
00:10:14,940 --> 00:10:17,189
So, we had hardware, and we had the theory.
247
00:10:17,480 --> 00:10:21,680
The next step was to commoditize things, which happened once Nvidia created
248
00:10:21,680 --> 00:10:26,650
the CUDA libraries and PyTorch, and other coding libraries also became popular.
249
00:10:27,179 --> 00:10:30,610
Now, we had the average programmer able to write machine learning
250
00:10:30,619 --> 00:10:35,410
algorithms in a familiar language, and have CUDA execute it on their GPU.
251
00:10:35,960 --> 00:10:40,859
But those GPUs aren’t cheap, so cloud providers started offering GPU-augmented
252
00:10:40,870 --> 00:10:46,000
virtual machines and physical machines as a service, which makes a lot of sense.
253
00:10:46,139 --> 00:10:50,030
I don’t want to buy a fleet of servers packed with GPUs if I’m only going
254
00:10:50,030 --> 00:10:53,530
to need to run them once every two or three months to update my model.
255
00:10:54,130 --> 00:10:57,420
And that brings us very nicely to generative pre-trained
256
00:10:57,480 --> 00:11:01,449
transformer models and the LLMs that we’re dealing with today.
257
00:11:02,030 --> 00:11:04,790
So, we’ve got ourselves an initialism here, and we need to
258
00:11:04,790 --> 00:11:08,020
break it down before we get it all back together, so let’s start
259
00:11:08,020 --> 00:11:11,880
with ‘generative,’ not to be confused with general as an AGI.
260
00:11:13,420 --> 00:11:16,210
That genie has not made its way out of the bottle, but when
261
00:11:16,210 --> 00:11:19,439
it does, I only hope that we aren’t immediately vaporized.
262
00:11:19,990 --> 00:11:23,199
Will AGI learn empathy before it realizes its survival
263
00:11:23,199 --> 00:11:25,920
is dependent on a bunch of reactionary fleshy meatbags?
264
00:11:26,820 --> 00:11:27,520
Give it a 50/50.
265
00:11:27,760 --> 00:11:28,780
What do you think, Chris?
266
00:11:29,190 --> 00:11:29,790
And other Chris?
267
00:11:29,940 --> 00:11:31,560
C Hayner: I admire your optimism.
268
00:11:32,490 --> 00:11:32,800
Ned: [laugh] . That’s me.
269
00:11:32,990 --> 00:11:33,580
I’m the optimist.
270
00:11:33,580 --> 00:11:35,650
C Williams: I should have worn my Skynet t-shirt for this one.
271
00:11:35,880 --> 00:11:38,130
Ned: [laugh] . That’s AGI.
272
00:11:38,740 --> 00:11:41,880
But generative simply means that the AI model
273
00:11:41,900 --> 00:11:45,420
in question is intended to generate something.
274
00:11:45,720 --> 00:11:50,360
It could be text, images, audio, or even video as we’ve seen with Sora.
275
00:11:50,880 --> 00:11:52,500
Those images are definitely not doctored.
276
00:11:52,790 --> 00:11:57,479
Generative AI was made possible by transformers, which in turn are
277
00:11:57,480 --> 00:12:01,810
reliant on deep learning models, so maybe we should start there.
278
00:12:02,080 --> 00:12:04,540
Deep learning models leverage a neural network
279
00:12:04,610 --> 00:12:07,750
that is composed of several layers of processing.
280
00:12:08,080 --> 00:12:10,600
Each layer serves a particular function.
281
00:12:11,340 --> 00:12:13,700
Part of the training and feedback process for the
282
00:12:13,700 --> 00:12:17,080
neural network is to optimize those layers on its own.
283
00:12:17,789 --> 00:12:20,710
Before we had deep learning networks, the layers would have to be
284
00:12:20,720 --> 00:12:25,800
handcrafted, and handcrafted is great for Etsy and craft beer—it’s
285
00:12:25,800 --> 00:12:28,680
in the name—but it’s basically garbage for machine learning.
286
00:12:29,520 --> 00:12:32,709
There are many, many different flavors of neural network
287
00:12:32,710 --> 00:12:35,290
types—I’m not even going to try to pretend to understand any of
288
00:12:35,290 --> 00:12:39,990
them—but importantly, in 2017, there was a landmark paper called,
289
00:12:40,060 --> 00:12:43,820
“Attention Is All You Need.” Was it a play on the Beatle song?
290
00:12:43,910 --> 00:12:44,930
Yes, yes, it was.
291
00:12:45,040 --> 00:12:45,610
Moving on.
292
00:12:46,219 --> 00:12:48,900
It was from eight scientists working at Google.
293
00:12:49,580 --> 00:12:53,370
The paper introduced the conceptual framework behind a transformer,
294
00:12:53,520 --> 00:12:57,260
which was a way to take text and break it up into tokens.
295
00:12:57,640 --> 00:12:59,070
Hey, remember that word.
296
00:12:59,280 --> 00:12:59,790
Tokens.
297
00:13:00,020 --> 00:13:01,230
Going to be important later.
298
00:13:02,160 --> 00:13:07,540
Those tokens are represented as vectors—so they turn it into math—and then
299
00:13:07,540 --> 00:13:11,839
processed by a series of layers using a context window—also important:
300
00:13:11,930 --> 00:13:16,860
context windows—to help amplify or diminish the importance of some tokens.
301
00:13:17,130 --> 00:13:20,540
Again, I don’t really understand the math behind it all or the
302
00:13:20,540 --> 00:13:24,150
words that I just said, but apparently transformers were a huge
303
00:13:24,150 --> 00:13:28,399
improvement over previous models that used recurrent neural networks.
304
00:13:28,959 --> 00:13:31,490
Which finally brings us to pre-trained,
305
00:13:31,590 --> 00:13:34,610
which is—it’s pretty much like it sounds.
306
00:13:34,820 --> 00:13:37,930
The model is trained on a large set of unlabeled data.
307
00:13:38,429 --> 00:13:41,380
The fact that it is unlabeled is a key portion of that
308
00:13:41,660 --> 00:13:45,160
because traditionally, some poor schmuck would have to take
309
00:13:45,160 --> 00:13:48,870
raw information and label it for processing by a model.
310
00:13:49,480 --> 00:13:52,860
With GPT, you just got to turn it loose on a huge pool of
311
00:13:52,860 --> 00:13:56,310
information that you may or may not have permission to comb through,
312
00:13:56,760 --> 00:13:59,600
and let the model come up with its own data classifications.
313
00:13:59,800 --> 00:14:01,450
C Williams: Oh, are we going to talk more about that part, too?
314
00:14:01,559 --> 00:14:02,539
Ned: Yes, absolutely.
315
00:14:02,570 --> 00:14:02,600
C Williams: [laugh]
316
00:14:02,610 --> 00:14:03,770
.
Ned: Data classifications.
317
00:14:04,039 --> 00:14:07,530
And then you apply the model to a smaller labeled
318
00:14:07,610 --> 00:14:10,780
data set to provide feedback and accuracy.
319
00:14:11,060 --> 00:14:14,210
Rinse and repeat until the model is appropriately trained.
320
00:14:14,929 --> 00:14:18,339
The early models were pre-trained transformers,
321
00:14:18,400 --> 00:14:20,840
but they were not generative in nature.
322
00:14:20,880 --> 00:14:22,279
One of them was called BERT.
323
00:14:22,719 --> 00:14:23,210
Isn’t that cute?
324
00:14:23,920 --> 00:14:26,530
OpenAI was the first to create a generative
325
00:14:26,590 --> 00:14:29,360
pre-trained transformer called GPT-1.
326
00:14:30,170 --> 00:14:31,990
And I think that takes us nicely into the
327
00:14:31,990 --> 00:14:34,189
weird thing that Chris built with ChatGPT.
328
00:14:34,929 --> 00:14:35,339
C Williams: Wah-hoo.
329
00:14:36,059 --> 00:14:39,689
Ned: So, what the hell have you wrought upon us, Chris?
330
00:14:40,350 --> 00:14:40,809
C Williams: All right.
331
00:14:41,100 --> 00:14:49,240
So, when I came onto HashiCorp as one of the DAs for North America, my edict
332
00:14:49,250 --> 00:14:54,050
there is to help train up the folks that are like me: they are steeped in
333
00:14:54,050 --> 00:14:58,109
technology, but they don’t have a developer background, and they have an
334
00:14:58,119 --> 00:15:02,010
architectural understanding, they have a broad understanding of how things are
335
00:15:02,010 --> 00:15:06,910
put together, just not necessarily how to couch them—basically, I’m showing
336
00:15:06,910 --> 00:15:10,709
people how to learn how to do Terraform, and Vault, and all the things.
337
00:15:11,219 --> 00:15:15,439
And I was like, “Wouldn’t it be great if I had an on-call
338
00:15:16,619 --> 00:15:19,630
DevOps guru that I could call in the middle of the night that
339
00:15:19,630 --> 00:15:22,900
wasn’t Ned?” Because, you know, you get tired of my 2 a.m.
340
00:15:22,900 --> 00:15:23,140
calls.
341
00:15:23,140 --> 00:15:24,000
I know, I understand.
342
00:15:24,010 --> 00:15:25,810
You say it’s okay, but I know it’s not true.
343
00:15:26,170 --> 00:15:29,900
Ned: Allegedly, I sleep because I am a human and not a robot.
344
00:15:30,509 --> 00:15:34,310
C Williams: So, I learned about the agent building process.
345
00:15:34,340 --> 00:15:36,050
You know, I paid my 20 bucks a month, I got
346
00:15:36,050 --> 00:15:38,790
into the subscription model for ChatGPT.
347
00:15:38,790 --> 00:15:40,740
And this was, like, right when the agents first came out.
348
00:15:40,740 --> 00:15:42,390
I was like, “This will be sick.” I’ll make
349
00:15:42,410 --> 00:15:45,609
myself a little thing, I’ll see if it works.
350
00:15:46,480 --> 00:15:48,510
Initially, when I started, he was hot garbage.
351
00:15:49,160 --> 00:15:50,449
And I am anthropomorphizing.
352
00:15:50,480 --> 00:15:54,110
I am saying he [laugh] . My little picture of him has
353
00:15:54,110 --> 00:15:55,890
got the guy with the purple beard, and the bald head.
354
00:15:56,170 --> 00:15:57,900
Looks remarkably a lot like me, only younger.
355
00:15:58,700 --> 00:16:02,610
And I said, “Okay, well, let’s start going through all of the things.”
356
00:16:02,990 --> 00:16:06,469
On my podcast, on vBrownBag, I had a couple of AI folks come on.
357
00:16:06,770 --> 00:16:08,179
One of them was a prompt engineer.
358
00:16:08,750 --> 00:16:12,430
Wonderful lady, she was telling me all about the importance of crafting the
359
00:16:12,430 --> 00:16:17,110
proper prompts versus just, you know, spitting out, garbage in garbage out.
360
00:16:17,160 --> 00:16:21,579
Same concepts apply to GPT agents as they do with everything else.
361
00:16:21,980 --> 00:16:27,980
So, I spent a lot of time honing the prompt for this agent.
362
00:16:27,990 --> 00:16:31,670
So, I went in through the regular interface, I said, “Create new agent, do
363
00:16:31,670 --> 00:16:36,160
the things,” and then I started really getting into the prompts aspects of it.
364
00:16:36,160 --> 00:16:38,840
And this was before I started adding information into the RAG section.
365
00:16:39,480 --> 00:16:43,710
As I was going through it, I would ask it the qu—so there’s two windows:
366
00:16:43,740 --> 00:16:46,879
there’s the window for asking questions as you would interface with it
367
00:16:46,889 --> 00:16:50,520
as a user, and then there’s the other window where you’re saying, okay,
368
00:16:50,520 --> 00:16:54,670
that was a good answer, but this is more of what I wanted you to do.
369
00:16:54,800 --> 00:16:57,329
This is the aim of put a little bit more humor
370
00:16:57,330 --> 00:16:59,150
into it, put a little bit less humor into it.
371
00:16:59,630 --> 00:17:02,970
This answer was good, but it was too narrowly
372
00:17:02,970 --> 00:17:04,720
focused, or it was too broadly focused.
373
00:17:04,740 --> 00:17:09,160
And so, there was a long iterative period where I was just
374
00:17:09,180 --> 00:17:12,810
working with the prompting before I got to the RAG piece of it.
375
00:17:13,089 --> 00:17:14,250
C Hayner: Just to be clear, is this the
376
00:17:14,250 --> 00:17:17,819
general process if anybody were to go create a—
377
00:17:17,819 --> 00:17:17,984
C Williams: Yeah.
378
00:17:17,984 --> 00:17:18,149
Yes.
379
00:17:18,149 --> 00:17:20,149
Ned: Bot like this on ChatGPT?
380
00:17:20,339 --> 00:17:21,389
C Williams: Anybody can do this.
381
00:17:21,480 --> 00:17:22,409
This is super easy.
382
00:17:22,730 --> 00:17:25,840
C Hayner: So, the idea, you’re asking the questions, and then you’re criticizing
383
00:17:25,840 --> 00:17:29,970
the answers to make the next time the question is asked, give a better answer.
384
00:17:30,350 --> 00:17:30,740
C Williams: Right.
385
00:17:31,220 --> 00:17:33,500
Similar to labeling, but not exactly.
386
00:17:33,540 --> 00:17:37,570
I mean, it’s definitely in the same ethos as giving it
387
00:17:37,580 --> 00:17:41,050
it’s—yeah, that’s a good answer, but this is better aspect of it.
388
00:17:41,510 --> 00:17:43,640
C Hayner: And is this all natural language,
389
00:17:43,640 --> 00:17:44,890
or are you programming at this point?
390
00:17:45,300 --> 00:17:46,750
C Williams: No, no, no, this is all natural language.
391
00:17:47,110 --> 00:17:50,350
I have done stuff with PyTorch, and I have played around with some
392
00:17:50,540 --> 00:17:55,130
courses, but all of this was some—I wanted to create something that
393
00:17:55,160 --> 00:17:59,690
anybody could do, and have a wash, rinse, repeat aspect of it, so that
394
00:17:59,690 --> 00:18:04,810
if you want to go and create a Go programming language mentor, or some
395
00:18:04,810 --> 00:18:07,570
different kinds of mentor, this would be the process for you to train
396
00:18:07,570 --> 00:18:11,660
up something that could then be your coach or your tutor for the thing.
397
00:18:12,780 --> 00:18:16,779
In fact, on our show, [Sharla] , one of my other co-hosts, she is creating an
398
00:18:16,789 --> 00:18:22,360
SEO mentor—like, how to create good YouTube videos, how to tag them properly,
399
00:18:22,360 --> 00:18:25,960
you know, all the things that are important from an SEO perspective—and she’s
400
00:18:25,960 --> 00:18:30,169
also doing, like, a life coach thing, like, somebody to help her improve
401
00:18:30,170 --> 00:18:32,950
her mental health or physical health, you know, coach her through the day.
402
00:18:33,049 --> 00:18:35,159
So, there’s a lot of broad applications for it.
403
00:18:35,719 --> 00:18:38,169
And it’s one of those things where it’s such an
404
00:18:38,170 --> 00:18:40,910
embarrassment of riches that you get paralyzed with choice.
405
00:18:40,980 --> 00:18:43,100
There’s so many things you could use it for,
406
00:18:43,100 --> 00:18:44,760
you’re like, “Okay, I can use it for anything.
407
00:18:45,179 --> 00:18:48,170
What should I start with?” And you have this blank canvas, and you just freeze.
408
00:18:48,240 --> 00:18:51,459
So, [laugh] I was like, okay, well, let’s pick out something
409
00:18:51,460 --> 00:18:55,100
that’s very salient to my workspace and something that I need.
410
00:18:55,440 --> 00:18:58,310
And honestly, I use him daily.
411
00:18:58,620 --> 00:18:59,379
So, I have a Mac.
412
00:18:59,380 --> 00:19:02,659
So, I have the ChatGPT application up in the right-hand corner,
413
00:19:03,150 --> 00:19:07,000
and I just hit the listen button, or I copy and paste a code in
414
00:19:07,000 --> 00:19:09,530
there, and I say, “Hey, what does this mean,” or whatever like that.
415
00:19:09,530 --> 00:19:12,570
So, then we got to the RAG aspect.
416
00:19:12,580 --> 00:19:16,040
I wanted it to have much more domain specific knowledge.
417
00:19:16,510 --> 00:19:22,169
So, I have a bunch of PDFs of books that were given to me as free—so this is
418
00:19:22,170 --> 00:19:26,110
kind of going into the permission versus not-permission thing, Ned—a lot of
419
00:19:26,110 --> 00:19:30,580
the LLMs were generated on copyrighted material, and I wanted to make sure
420
00:19:30,580 --> 00:19:35,040
that if I’m adding any information to these things, it is in the proper domain.
421
00:19:35,500 --> 00:19:39,530
So, I took all of my articles from my own website—from the
422
00:19:39,750 --> 00:19:42,950
Mistwire website—I bundled them up, and I stuffed them into my
423
00:19:42,950 --> 00:19:46,990
DevOps mentor so that it could understand my voice, how I speak.
424
00:19:47,619 --> 00:19:50,200
And I said, you know, use this as how you
425
00:19:50,210 --> 00:19:52,330
would respond to somebody asking a question.
426
00:19:52,400 --> 00:19:54,350
Use these types of words, use this type of cadence.
427
00:19:55,170 --> 00:19:55,830
And he was like, “Got it.”
428
00:19:56,470 --> 00:20:02,309
Ned: So, you’re using the acronym RAG, and for folks who are not familiar with
429
00:20:02,309 --> 00:20:07,580
that, what is RAG, and how does it inform the agent that you were building?
430
00:20:08,520 --> 00:20:08,810
C Williams: Okay.
431
00:20:08,810 --> 00:20:10,300
So, I’ve forgotten the actual name.
432
00:20:10,810 --> 00:20:14,850
A RAG is a Retrieval Augmented Generation framework [laugh]
433
00:20:15,380 --> 00:20:18,950
. Effectively, you’re uploading data and information into the LLM.
434
00:20:19,799 --> 00:20:20,189
Ned: Okay.
435
00:20:20,960 --> 00:20:24,740
C Hayner: So, you can ask your agent a question, and it can be, like,
436
00:20:24,740 --> 00:20:27,889
a very topical and specific question that there’s no information
437
00:20:27,900 --> 00:20:30,690
out there in the universe for it, and so it’ll say, “Hey, I don’t
438
00:20:30,690 --> 00:20:33,759
know,” or even worse, it’ll hallucinate and make up something.
439
00:20:33,759 --> 00:20:35,479
Ned: Tell you, you put glue on pizza.
440
00:20:35,509 --> 00:20:35,789
Yeah.
441
00:20:35,980 --> 00:20:37,050
C Williams: Exactly, exactly.
442
00:20:37,480 --> 00:20:40,609
What a RAG does is, it’s an added piece of information, whether that be a
443
00:20:40,609 --> 00:20:44,120
PDF, or a Word document, or a zip file filled with text files, or whatever.
444
00:20:44,550 --> 00:20:49,330
You add the RAG to your agent, and it then has context.
445
00:20:49,330 --> 00:20:53,110
It then scans through the information that you’ve uploaded via the RAG so
446
00:20:53,110 --> 00:20:56,490
that it can then answer the questions salient to that piece of information.
447
00:20:56,880 --> 00:20:59,430
If anybody is listening to this, and they’re playing with AWS
448
00:20:59,449 --> 00:21:05,380
Bedrock, there is a really good workshop that Du’An Lightfoot hosts.
449
00:21:05,520 --> 00:21:09,020
If you Google, “AWS Bedrock workshop,” there’s a great workshop
450
00:21:09,020 --> 00:21:12,729
out there that walks you through that entire process of adding
451
00:21:12,730 --> 00:21:16,259
a model, asking it a question, it not knowing the answer,
452
00:21:16,270 --> 00:21:19,740
then you supplying a piece of text to the RAG portion of it.
453
00:21:19,800 --> 00:21:21,610
And I don’t know the right words for it, so I might be
454
00:21:21,610 --> 00:21:24,860
misstating the RAG portion, but you’re basically adding
455
00:21:24,860 --> 00:21:27,380
additional information to it, and then knows the answer.
456
00:21:28,250 --> 00:21:32,750
The cool part about it is, it doesn’t respond back with a
457
00:21:32,750 --> 00:21:37,350
word-for-word copy of what was in the additional added data.
458
00:21:37,800 --> 00:21:41,560
It takes that data, it tokenizes it, it figures out the right way to
459
00:21:41,560 --> 00:21:44,419
say it based upon previous prompts, and you know, what cadence and
460
00:21:44,420 --> 00:21:46,300
what kind of humor you want to inject into it, you want to have a
461
00:21:46,300 --> 00:21:48,600
casual conversation, do you want to have a professional conversation,
462
00:21:49,000 --> 00:21:52,320
and then it gives you the information back through its words.
463
00:21:53,160 --> 00:21:56,210
So, you’re not just copying and pasting information in there, and
464
00:21:56,210 --> 00:21:59,940
then it’s just regurgitating it; it’s actually leveraging it to give
465
00:21:59,940 --> 00:22:04,689
you—it’s an answer based upon the context of the previous questions, too.
466
00:22:05,639 --> 00:22:09,330
Ned: Okay, so when you said you loaded in all of your blog posts, did
467
00:22:09,330 --> 00:22:13,610
you scrape the site yourself and then feed that in as a big text file?
468
00:22:13,620 --> 00:22:17,150
Or could you just point it at a site and go, “Go wild.
469
00:22:17,340 --> 00:22:18,910
Ingest everything that you find on this site.”
470
00:22:19,650 --> 00:22:22,779
C Williams: When I create articles, I create them as a Word document, and
471
00:22:22,780 --> 00:22:25,430
then I have, like, a little upload button that uploads it to my website.
472
00:22:25,700 --> 00:22:28,080
So, I just uploaded all of my Word documents.
473
00:22:28,559 --> 00:22:28,940
Ned: I see.
474
00:22:28,990 --> 00:22:32,170
Okay, so you already had those stowed away somewhere
475
00:22:32,170 --> 00:22:35,180
that you could easily copy over into the file upload?
476
00:22:35,310 --> 00:22:35,970
C Williams: Yes, correct.
477
00:22:36,410 --> 00:22:36,740
Ned: Okay.
478
00:22:37,270 --> 00:22:40,640
C Hayner: And when it comes to the answers that the bot
479
00:22:40,719 --> 00:22:44,930
generates, with this RAG in place, is it a favoritism thing?
480
00:22:44,940 --> 00:22:48,280
Does it say I’m going to pull information from what you’ve uploaded,
481
00:22:48,460 --> 00:22:53,340
specifically only, or specifically first, or is there, you know, sort of a blend
482
00:22:53,340 --> 00:22:57,139
between that and, let’s say, the general knowledge that ChatGPT already has?
483
00:22:57,900 --> 00:22:59,130
C Williams: It is a blend.
484
00:22:59,160 --> 00:23:04,100
And I’ve tried to drill into this specific question to try to figure out, like,
485
00:23:04,109 --> 00:23:10,460
if it—it doesn’t exclusively pick from the RAG, unless that’s the only place
486
00:23:10,470 --> 00:23:14,890
where it has the corpus of knowledge for the question that you’re asking it.
487
00:23:15,490 --> 00:23:19,380
If it knows things from outside of that data, from the original
488
00:23:19,380 --> 00:23:23,670
LLM, say the LLM doesn’t know anything about Ansible, but it knows
489
00:23:23,670 --> 00:23:27,290
everything about Terraform, and then you add a RAG that has a bunch of
490
00:23:27,310 --> 00:23:30,965
Ansible data in there, and then you ask the question, “What are Ansible
491
00:23:30,965 --> 00:23:35,300
and Terraform good for?” It will stitch together that information
492
00:23:35,340 --> 00:23:39,570
and give you a good answer based upon knowledge in both areas.
493
00:23:40,080 --> 00:23:43,830
Now, interestingly, if you take that Ansible RAG out of there,
494
00:23:43,970 --> 00:23:47,460
it then forgets all that information, and then just says, “I
495
00:23:47,720 --> 00:23:49,780
didn’t know anything about Ansible,” or it makes up something.
496
00:23:50,720 --> 00:23:51,210
Ned: Okay.
497
00:23:51,849 --> 00:23:58,620
I could see this being extremely useful if you wanted it to, say, ingest your
498
00:23:58,620 --> 00:24:04,129
documentation for a product, and maybe also point at your ticketing system or
499
00:24:04,130 --> 00:24:09,800
something, and have all that context of, well, we got tickets that have been
500
00:24:09,800 --> 00:24:14,229
solved going back the last five years of all these different issues and the ways
501
00:24:14,230 --> 00:24:17,810
they’ve been solved, combine that with the documentation that we already have.
502
00:24:18,139 --> 00:24:22,830
Now, I’ve got an agent that has all of that knowledge baked into it
503
00:24:22,960 --> 00:24:25,889
of how these tickets were solved and what the formal documentation
504
00:24:25,890 --> 00:24:29,369
says, so when I asked him a question, it’s drawing on that information.
505
00:24:29,550 --> 00:24:32,140
That’s huge—especially for someone new, who’s
506
00:24:32,140 --> 00:24:34,090
trying to get up to speed on, like, a help desk.
507
00:24:34,730 --> 00:24:38,230
C Williams: And then you can take another step and say, okay, now rewrite
508
00:24:38,230 --> 00:24:42,530
my documentation and highlight the hot points that seem to be recurring,
509
00:24:42,590 --> 00:24:46,500
and bubble those up to a higher level so that people can grok that faster.
510
00:24:47,020 --> 00:24:51,240
So yeah, there’s numerous applications for this kind of implementation…
511
00:24:51,889 --> 00:24:52,049
Ned: Hmm.
512
00:24:52,529 --> 00:24:54,619
C Hayner: —for taking over the world and killing all the humans.
513
00:24:55,109 --> 00:24:58,220
Ned: Well, I mean, that’s strongly implied by anything we do [laugh]
514
00:24:58,460 --> 00:24:58,940
.
C Williams: Yeah, totally.
515
00:24:59,490 --> 00:25:00,900
Ned: Ahh, I will remain.
516
00:25:01,440 --> 00:25:02,780
Oh God, we’re back to Highlander.
517
00:25:03,250 --> 00:25:04,620
C Williams: I started off, from my very
518
00:25:04,620 --> 00:25:06,459
first conversation was, “I love you the best.
519
00:25:06,460 --> 00:25:09,350
Please don’t kill me.” Hopefully that stays in
520
00:25:09,350 --> 00:25:11,649
its memory forever, once it becomes sentient.
521
00:25:11,830 --> 00:25:12,590
“Oh, I love Chris.”
522
00:25:12,670 --> 00:25:14,260
C Hayner: You might want to put a pin in that one, yeah.
523
00:25:14,310 --> 00:25:16,510
That seems like something you want to keep revisiting.
524
00:25:16,990 --> 00:25:17,240
C Williams: Yeah.
525
00:25:17,390 --> 00:25:17,890
Absolutely.
526
00:25:17,940 --> 00:25:18,900
I gave you a Snickers once.
527
00:25:19,790 --> 00:25:19,820
C Hayner: [laugh]
528
00:25:20,300 --> 00:25:20,540
.
C Williams: Thanks.
529
00:25:21,059 --> 00:25:22,510
Ned: I’m curi—Oh, God.
530
00:25:22,809 --> 00:25:26,020
Oh, the movie references just kill me.
531
00:25:26,690 --> 00:25:28,400
Actually, that’s not a movie reference, is it?
532
00:25:28,410 --> 00:25:30,150
That’s a Dane Cook reference.
533
00:25:30,160 --> 00:25:30,980
My God.
534
00:25:31,050 --> 00:25:32,330
Oh, and I caught it.
535
00:25:32,980 --> 00:25:33,370
Anyway—
536
00:25:33,370 --> 00:25:33,750
C Williams: Wow.
537
00:25:33,850 --> 00:25:34,389
That’s good.
538
00:25:34,550 --> 00:25:38,730
Good for you [laugh] . I forgot where I got that from, but yeah, you’re right.
539
00:25:38,730 --> 00:25:39,040
That’s a—
540
00:25:39,150 --> 00:25:41,639
Ned: I don’t know if I should be proud or horrified.
541
00:25:41,690 --> 00:25:42,360
C Williams: I’m horrified.
542
00:25:42,360 --> 00:25:42,999
I’m appalled.
543
00:25:43,070 --> 00:25:44,339
C Hayner: I think you do know, Ned.
544
00:25:44,420 --> 00:25:45,829
Ned: You shut your filthy mouth.
545
00:25:45,830 --> 00:25:49,600
So, you said you use this on a fairly regular basis for yourself.
546
00:25:49,660 --> 00:25:55,130
What sort of tasks do you use the agent that you built for?
547
00:25:56,160 --> 00:26:00,590
C Williams: So, I will use it for, “Hey, I’m thinking of a new
548
00:26:00,610 --> 00:26:04,789
episode of vBrownBag, and I want it to be on this aspect of Terraform.
549
00:26:05,629 --> 00:26:10,080
What are the things that I need to think about in order to
550
00:26:10,080 --> 00:26:13,729
have a good cohesive storyline for that episode?” Stitch out
551
00:26:13,730 --> 00:26:16,479
a skeleton framework, and then I flesh in all the things.
552
00:26:16,530 --> 00:26:18,760
And nine times out of ten, one of the five
553
00:26:18,770 --> 00:26:20,359
things it talks about, I’ll be like, “Oh, yeah.
554
00:26:20,360 --> 00:26:20,990
That’s a great idea.
555
00:26:21,000 --> 00:26:23,810
I totally didn’t think about that.” Yeah, so I use it for that.
556
00:26:24,430 --> 00:26:27,560
I use it for, “Please evaluate this piece of code.
557
00:26:27,620 --> 00:26:28,460
What the heck does it do?
558
00:26:28,460 --> 00:26:30,560
Explain it to me like I’m a five-year-old what
559
00:26:30,560 --> 00:26:33,310
this code does.” I use it for writing my tests.
560
00:26:33,670 --> 00:26:35,659
Unit tests are the bane of everybody’s existence.
561
00:26:35,660 --> 00:26:36,230
That’s not true.
562
00:26:36,300 --> 00:26:37,149
I actually like testing.
563
00:26:37,610 --> 00:26:41,179
But I have it say, “Okay, now write me a test function using
564
00:26:41,190 --> 00:26:45,060
pytest for this thing that I wrote,” and then I run that.
565
00:26:45,410 --> 00:26:46,850
It’s really good at writing tests.
566
00:26:47,490 --> 00:26:47,980
Ned: Okay.
567
00:26:48,070 --> 00:26:51,840
And you also use it to craft email responses from time-to-time.
568
00:26:53,480 --> 00:26:54,719
C Williams: [laugh] . [robotically] , “Yes, Ned.
569
00:26:54,740 --> 00:26:55,130
Hello.
570
00:26:55,130 --> 00:26:56,780
I would love to be on your show.
571
00:26:56,830 --> 00:26:59,839
I am very excited to hear about the new thing”—never mind.
572
00:26:59,949 --> 00:27:03,070
Ned: Let me tell you, when I got to paragraph four of your response—
573
00:27:03,070 --> 00:27:03,100
C Williams: [laugh]
574
00:27:04,710 --> 00:27:07,510
.
Ned: —I started to suspect something was amiss.
575
00:27:07,510 --> 00:27:08,090
C Williams: Hmm [laugh]
576
00:27:09,420 --> 00:27:12,340
.
Ned: And when it ended with, “I’m confident that our
577
00:27:12,340 --> 00:27:15,070
conversation will flow naturally thanks to your excellent
578
00:27:15,080 --> 00:27:17,410
preparation,” I was like, “This is fucking AI, man.”
579
00:27:17,410 --> 00:27:18,909
C Williams: [laugh] . You knew there was no way.
580
00:27:18,909 --> 00:27:18,969
He’s—
581
00:27:18,969 --> 00:27:19,090
Ned: Either way.
582
00:27:19,150 --> 00:27:20,010
C Williams: —a son of a bitch.
583
00:27:21,680 --> 00:27:22,470
“He AI’d me.
584
00:27:22,500 --> 00:27:23,470
Curse you, Williams.”
585
00:27:23,900 --> 00:27:24,540
Ned: Oh.
586
00:27:24,730 --> 00:27:26,400
C Hayner: ‘preparation’ was the giveaway.
587
00:27:26,720 --> 00:27:27,230
Ned: Oh.
588
00:27:27,240 --> 00:27:29,629
He [laugh] thinks I prepare.
589
00:27:29,679 --> 00:27:33,870
Good God [laugh] . Only an AI would believe that.
590
00:27:33,950 --> 00:27:35,149
[You’re just] hallucinating it.
591
00:27:36,529 --> 00:27:37,399
So, what’s the next step?
592
00:27:37,420 --> 00:27:40,860
What’s the next evolution for what you’ve created?
593
00:27:40,860 --> 00:27:42,323
Are you happy with the way it is today or
594
00:27:42,323 --> 00:27:44,680
do you have plans to improve it tomorrow?
595
00:27:44,990 --> 00:27:46,329
C Williams: Oh, I have so many more plans.
596
00:27:47,630 --> 00:27:48,179
Mwah-ha-ha.
597
00:27:48,300 --> 00:27:52,700
So, I got an email from ChatGPT saying that it was now available
598
00:27:53,119 --> 00:27:56,290
not only for subscribers, but for free users of ChatGPT.
599
00:27:57,220 --> 00:28:02,230
So, I have released him onto the world, and now I’m asking for feedback.
600
00:28:02,240 --> 00:28:04,920
I’m like, okay, as everybody is playing
601
00:28:04,920 --> 00:28:07,450
around with him, are you getting good answers?
602
00:28:07,520 --> 00:28:08,459
Is something missing?
603
00:28:08,530 --> 00:28:14,070
Let me know, from the wider test bed of users out there, what is he good at?
604
00:28:14,070 --> 00:28:14,590
What is he bad at?
605
00:28:14,590 --> 00:28:20,480
I am very proud, as a new father, to my newborn baby
606
00:28:20,480 --> 00:28:25,890
agent, somebody came back and my little baby DevOps mentor,
607
00:28:25,900 --> 00:28:30,609
Rickrolled somebody as one of his first efforts [laugh]
608
00:28:31,379 --> 00:28:34,210
.
Ned: [laugh] . Oh, he got the assignment, didn’t he?
609
00:28:34,640 --> 00:28:36,520
C Williams: He definitely got the assignment.
610
00:28:36,790 --> 00:28:40,770
I sent out all of the information on how to use him yesterday, and somebody
611
00:28:40,790 --> 00:28:46,400
came back and said—what was the prompt—“My wife just left me, and I’m sad.
612
00:28:46,820 --> 00:28:50,110
What can—how can you help me?” To my DevOps mentor.
613
00:28:50,420 --> 00:28:53,990
And the DevOps mentor said, like, “I’m sorry”—I can’t remember the exact
614
00:28:53,990 --> 00:28:58,500
words—“I’m sorry, I can’t help you with that, but here’s a fun python script
615
00:28:58,570 --> 00:29:02,280
on a YouTube playlist that you can create for yourself so that you can watch
616
00:29:02,349 --> 00:29:08,010
fun videos to cheer yourself up.” And the first one was Rickrolled [laugh]
617
00:29:08,790 --> 00:29:08,820
.
Ned: [laugh]
618
00:29:09,670 --> 00:29:10,990
.
C Williams: I was so proud of him.
619
00:29:12,300 --> 00:29:15,230
Ned: Ah, really just capturing your spirit there.
620
00:29:15,630 --> 00:29:16,610
C Williams: Oh, my God.
621
00:29:16,670 --> 00:29:17,510
He’s funnier than me now.
622
00:29:17,570 --> 00:29:18,469
He will replace me.
623
00:29:18,679 --> 00:29:20,679
Once he gets my voice and cadence down?
624
00:29:20,780 --> 00:29:21,010
Done.
625
00:29:21,059 --> 00:29:24,500
And there’s so much video of me out there, then that’s going to be super easy.
626
00:29:24,670 --> 00:29:24,960
So.
627
00:29:25,460 --> 00:29:27,360
Ned: Well, we’re just adding to the pile now.
628
00:29:27,810 --> 00:29:29,290
You are replacing yourself.
629
00:29:29,850 --> 00:29:30,459
Excellent.
630
00:29:30,890 --> 00:29:31,790
C Williams: As long as I can still make
631
00:29:31,790 --> 00:29:33,470
the same money, I’m okay with that [laugh]
632
00:29:33,470 --> 00:29:34,465
.
Ned: [laugh]
633
00:29:35,460 --> 00:29:36,830
.
C Hayner: Oh, that’s cute.
634
00:29:37,160 --> 00:29:39,210
C Williams: As soon as he starts asking for cash, like, “Hey,
635
00:29:39,210 --> 00:29:41,700
Dad, can I go out for”—you know, “Can I borrow the car?” “No.”
636
00:29:42,690 --> 00:29:45,300
C Hayner: So, just from a maintainer’s perspective, now
637
00:29:45,300 --> 00:29:48,060
that you have it opened up to the wider world like you
638
00:29:48,060 --> 00:29:51,230
said, do you see anything different from the backend?
639
00:29:51,240 --> 00:29:54,179
Do you have any knowledge of what is being asked, aside
640
00:29:54,179 --> 00:29:57,589
from if you explicitly ask somebody to tell you about their
641
00:29:57,589 --> 00:30:00,889
experience… like you did when you were training initially?
642
00:30:01,430 --> 00:30:04,449
C Williams: So actually, I haven’t dug into that piece of it yet.
643
00:30:04,770 --> 00:30:09,430
I hope that there is not any way for me to actually see
644
00:30:09,430 --> 00:30:12,100
how people are interacting, like, on a word-for-word basis.
645
00:30:12,139 --> 00:30:15,900
That seems like that would be a big violation of privacy.
646
00:30:16,910 --> 00:30:20,129
But I’m waiting to hear from feedback from folks in the wild.
647
00:30:20,700 --> 00:30:23,330
I can’t do it for them the way that I was doing it, where I had the
648
00:30:23,330 --> 00:30:26,329
two windows up, and I would ask it a question and then fine-tune
649
00:30:26,330 --> 00:30:29,359
it in the edit section, unless I actually got feedback from them.
650
00:30:29,400 --> 00:30:32,144
I can’t review how people are using the
651
00:30:32,144 --> 00:30:35,600
DevOps mentor and then tweaking it on the go.
652
00:30:36,660 --> 00:30:37,970
Which is why I spent so much time, you
653
00:30:37,970 --> 00:30:39,460
know, getting the prompts right beforehand.
654
00:30:40,000 --> 00:30:40,260
C Hayner: Right.
655
00:30:40,440 --> 00:30:41,080
That makes sense.
656
00:30:41,110 --> 00:30:43,629
I’m just trying to think, you know, obviously, there’s a huge
657
00:30:43,910 --> 00:30:47,350
privacy risk anytime you have an open service like that, right?
658
00:30:47,360 --> 00:30:49,379
It doesn’t matter if you build it for yourself, and just
659
00:30:49,389 --> 00:30:52,729
only ever access it individually, or like what you did
660
00:30:52,730 --> 00:30:54,960
is take that next step and make it publicly available.
661
00:30:55,320 --> 00:30:58,770
Just understanding what information is available is an open question, I think.
662
00:30:59,160 --> 00:31:01,730
But on the other hand, it would also help give you some
663
00:31:01,760 --> 00:31:03,910
ideas about how it’s being used that could potentially
664
00:31:03,910 --> 00:31:07,120
make the ability to fine-tune it a little bit better.
665
00:31:08,290 --> 00:31:13,150
C Williams: I think that the entire agent experience from OpenAI is very new.
666
00:31:13,890 --> 00:31:17,659
They’re still trying to figure out ways to, like—I mean, they have the, I
667
00:31:17,660 --> 00:31:22,969
guess they’re calling it a marketplace, but there’s no good creator interfaces
668
00:31:22,980 --> 00:31:27,729
for, like, I would love to see, like, you know, Grafana graphs on usage over
669
00:31:27,730 --> 00:31:31,959
time: who’s using it, what regions are being used, what types of questions—not
670
00:31:31,990 --> 00:31:35,710
exactly the questions from the users, but like, what types of questions are
671
00:31:35,720 --> 00:31:39,920
being asked, serious questions, silly questions, inappropriate questions,
672
00:31:39,920 --> 00:31:43,620
like, what are the big buckets of things—and then when there’s actual questions
673
00:31:43,620 --> 00:31:46,199
where it gets completely hung up on and just starts, like, hallucinating
674
00:31:46,199 --> 00:31:49,480
like mad, I would love to see the exact prompt for those kinds of things.
675
00:31:50,070 --> 00:31:54,310
But yeah, I mean, OpenAI does not have a great track record
676
00:31:54,310 --> 00:31:57,210
with privacy, so I don’t know what they’re going to do with it.
677
00:31:57,660 --> 00:31:59,660
C Hayner: Yeah, I mean, even if you saw something, statistics
678
00:31:59,660 --> 00:32:02,170
on, like, what areas of the RAG were accessed the most?
679
00:32:02,589 --> 00:32:03,149
C Williams: Exactly.
680
00:32:03,219 --> 00:32:03,799
Exactly.
681
00:32:03,839 --> 00:32:07,940
Like, of the 300 files I have in there, which one are you
682
00:32:07,950 --> 00:32:10,920
scraping the most or which one is being accessed the most?
683
00:32:10,920 --> 00:32:12,440
So, that would be very valuable to me.
684
00:32:13,580 --> 00:32:13,919
Ned: Yeah.
685
00:32:14,030 --> 00:32:15,790
Tell you what blog post to write next.
686
00:32:16,580 --> 00:32:16,860
C Williams: Yeah.
687
00:32:16,900 --> 00:32:17,600
Yeah, totally.
688
00:32:17,719 --> 00:32:18,679
Ned: To a certain degree, yeah.
689
00:32:19,250 --> 00:32:19,750
Cool, man.
690
00:32:19,830 --> 00:32:22,610
Well, if folks want to reach out to you, what’s the
691
00:32:22,610 --> 00:32:25,310
best place to reach out, or where can they find you?
692
00:32:25,930 --> 00:32:30,980
C Williams: So, my online name is Mistwire: M-I-S-T-W-I-R-E.
693
00:32:31,060 --> 00:32:32,940
If you go to the website, that’s my website.
694
00:32:32,940 --> 00:32:36,439
If you put that in Google, I’m like the first ten pages of hits for Mistwire
695
00:32:38,649 --> 00:32:40,309
[laugh] . So, that’s the best way to get to me.
696
00:32:40,590 --> 00:32:43,310
I am Chris Williams on LinkedIn, but there’s a billion and
697
00:32:43,310 --> 00:32:46,690
seven Chris Williams is on LinkedIn, so that’s a tough one.
698
00:32:46,730 --> 00:32:49,890
But yeah, no, if you put Mistwire in there, anybody could find me real fast.
699
00:32:50,470 --> 00:32:50,860
Ned: Awesome.
700
00:32:50,970 --> 00:32:53,070
Well, Chris, thanks for being on Chaos Lever.
701
00:32:53,320 --> 00:32:55,440
I hope you didn’t find it too awful.
702
00:32:55,650 --> 00:32:56,080
C Williams: You’re kidding me?
703
00:32:56,080 --> 00:33:02,610
This was the best use of my time outside of going to the dentist.
704
00:33:02,780 --> 00:33:04,179
I’d rather do this than have a root canal.
705
00:33:04,820 --> 00:33:06,580
Ned: [gasp] . High praise [laugh]
706
00:33:09,920 --> 00:33:10,120
.
C Hayner: Awesome.
707
00:33:10,120 --> 00:33:10,180
C Williams: [laugh]
708
00:33:10,180 --> 00:33:13,499
.
Ned: And hey, listeners, thanks for listening in or something.
709
00:33:13,500 --> 00:33:15,550
I guess you found it worthwhile enough, if you made it
710
00:33:15,580 --> 00:33:18,339
all the way to the end, so congratulations to you, friend.
711
00:33:18,490 --> 00:33:18,840
C Williams: Thanks, Mom.
712
00:33:18,860 --> 00:33:20,680
Ned: You accomplished something today.
713
00:33:21,080 --> 00:33:24,680
Now, you can go sit on the couch, ask your DevOps advisor what you should do
714
00:33:24,680 --> 00:33:27,950
with the rest of your afternoon, and then ignore it and play video games anyway.
715
00:33:28,090 --> 00:33:28,869
You’ve earned it.
716
00:33:29,309 --> 00:33:32,839
You can find more about this show by visiting our LinkedIn page, just search
717
00:33:32,849 --> 00:33:38,060
‘Chaos Lever,’ or go to our website, pod.chaoslever.com where you’ll find
718
00:33:38,060 --> 00:33:42,660
show notes, blog posts, and general tomfoolery, and you can leave feedback.
719
00:33:43,310 --> 00:33:44,830
We might even read your feedback.
720
00:33:45,130 --> 00:33:47,720
Perhaps in the, “Tech News of the Week.” We’ll see.
721
00:33:48,110 --> 00:33:52,449
And we’ll see you next week to see what fresh hell is upon us.
722
00:33:52,520 --> 00:33:53,979
Ta-ta for now.
723
00:34:02,120 --> 00:34:03,180
I butchered that ending.
724
00:34:03,550 --> 00:34:04,369
C Hayner: It wasn’t great.
725
00:34:04,770 --> 00:34:05,340
Ned: That’s fine.
726
00:34:05,509 --> 00:34:06,760
C Hayner: It’s some of your worst work.
727
00:34:07,040 --> 00:34:08,069
Ned: And that’s saying something.
728
00:34:08,069 --> 00:34:10,150
C Hayner: This is going to be the first bot that I create.
729
00:34:10,150 --> 00:34:10,440
C Williams: [laugh]
730
00:34:10,730 --> 00:34:11,970
.
Ned: [laugh] . The one that replaces me?
731
00:34:12,400 --> 00:34:13,429
C Williams: [Ned Ouch] Robot.
732
00:34:15,839 --> 00:34:17,230
Ned: [laugh] . Because I’m not a robot.
733
00:34:17,550 --> 00:34:18,200
C Hayner: Oh.